Reflections on remote teaching during the COVID-19 pandemic
- Deborah Talmi
- Sep 22, 2022
- 4 min read
When I took responsibility for the move to teach remotely in my department, soon after lockdown was announced in March 2020, I knew that I lacked something quite essential: expertise in teaching. While academics in my role often had significant lecturing experience, and even some professional teaching recognition (for example, I am a fellow of the higher education academy), most of us were not education experts.
I was fortunate enough to have had access to seminars and workshops on online learning at my University; but the sessions, invaluable as they were, did not present either the theoretical or empirical evidence base for the recommendations they proffered. As a psychological scientist, I felt the gap particularly keenly. Not only was I used to being highly familiar with the theories and data that matter for my research work, but here the domain of the literature that underlay recommendations focused on human learning – a topic that is not a million miles away from my own expertise in memory and emotion. Yet it was entirely alien. And given the time pressure, I had no chance to master it.
What rescued me was a metaphor – one that I think could resonate with other scientists, especially those who are familiar with Bayesian statistics.
In a nutshell, the hierarchical predictive processing framework reconceptualised brain processes. Rather than bottom-up information processing, the brain is conceived as engaged in perfecting its models of the world. It uses sensory information to update its stored models, according to Bayes theorem; the more discrepant and unexpected the sensory data, the more the model changes to accommodate it. Internal models, in turn, predict what new sensory information would come next. The essential idea is that accurate internal models – those that reflect reality as it is and minimise surprise – are adaptive for survival. If your model predicts accurately where the stone your rival had thrown at you would land, you move more effectively to preserve your internal model of an intact body. Many cognitive neuroscientists use this framework to explain visual and auditory perception, and action control; I have used it in researching pain.
Strangely, I found that these abstract theories about how the brain works helped me understand the principles of effective teaching. There are, of course, many competing theories about how people learn and change, but for me, the computational principle of hierarchical predictive processing served as a useful metaphor, which helped me absorb the information that was available, and implement it in my Department. Given the pressures we were facing and continue to face I thought that this may also be useful for others, too.
Patrick Terenzini is renowned for his comprehensive review of students’ learning. His 50 years of scholarly work allowed him to distil the six essential characteristics of effective student experiences that lead to demonstrable success. With a Bayesian hat on, I could make more sense of the underlying reasons for the importance of these characteristics, and what relationship they bore to each other.
In predictive processing, we learn when our internal models change. Students come to lectures with a variety of internal models; taking an example from my own teaching, students have ideas about the relationship between feeling and thinking. For internal models to change, they must give rise to predictions that are inaccurate – that are challenged by subsequent information. Effective lecturers, therefore, would provide students with provocations that contradict their predictions (“educationally effective student experiences involve encounters with challenging ideas or people…”). For example, a student who believes that feelings can influence thought, but not the other way around, may be presented with an example where adopting a particular attitude changed subjective experience of negative affect, and even the associated physiological and neural correlates. A provocation is not enough on its own if it is only processed shallowly. To link the challenge to the model that needs to change, it needs to be processed at the appropriate level of the processing hierarchy (“educationally effective student experiences…Require students’ active engagement with the challenge”). While provocations could be delivered by lecturers, a single surprising experience will have a limited effect on entrenched models. The Bayesian approach therefore naturally explains why effective experiences “encourage active, real-world learning” and “involve other people”. These provide opportunities for students to use recently-updated internal models to make predictions and update their models until they become gradually more accurate.
The Bayesian framework also helped me think in a new way about the more pastoral side of teaching. Clinical psychologists have used ideas similar to the hierarchical predictive processing framework to explain the difficulty patients have when deeply-personal internal models are shaken up. The framework suggests that because it is so adaptive to predict upcoming stimulation, we all try to limit surprise. Yet instead of changing internal models, people can actively change the world around them in order to limit exposure to surprising information – for example, by forgetting material after the exam or even dropping out of a course. This insight could help us busy academics grasp why it is important that challenging learning “Occur in supportive environments”.
Terenzini’s principles, and their internal principles as reflected through the Bayesian metaphor, apply to the design of online learning in HE just as they do to any other form of learning, including the learning that is part of our academic research and professional development. A case in point was the challenge that the move to remote teaching provided for both students and academics. News from around the country suggested that remote teaching and learning could be hugely stressful. This motivated us to actively foster a supportive environment for the discussions that took place internally about remote teaching, and plan the process of change as a co-design through widespread staff and student consultation. Anecdotally, my sense is that despite the difficulties everyone faced, we maintained our trust in each other and the sense of working together collegiately. The Bayesian metaphor also helped us recognise that we can only improve our provision by allowing the reality on the ground to disconfirm our predictions. We took this forward through surveys of staff and students in November and December 2020, and were gratified when over half of the teaching staff the student body took the time to share their thoughts and ideas with us about how to make things better. There is clearly a lot more we can do – but we have the routes of a positive process to continue to learn together.
Thank you to: A. Greve, D. Good and I. Fay for comments on this text.
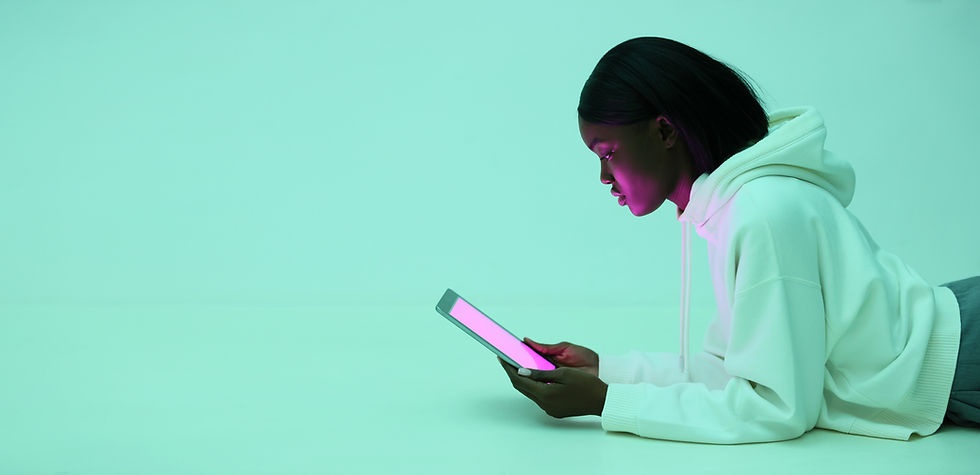
Comments